Nuclear receptor modulators: Catching information by machine learning
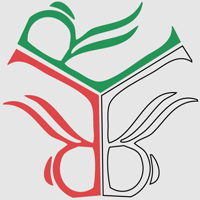
All claims expressed in this article are solely those of the authors and do not necessarily represent those of their affiliated organizations, or those of the publisher, the editors and the reviewers. Any product that may be evaluated in this article or claim that may be made by its manufacturer is not guaranteed or endorsed by the publisher.
Nuclear receptors (NRs) are involved in fundamental human health processes and are a relevant target for toxicological risk assessment. To help prioritize chemicals that can mimic natural hormones and be endocrine disruptors, computational models can be a useful tool.1,2 In this work we i) created an exhaustive collection of NR modulators and ii) applied machine learning methods to fill the data-gap and prioritize NRs modulators by building predictive models.
PAGEPress has chosen to apply the Creative Commons Attribution NonCommercial 4.0 International License (CC BY-NC 4.0) to all manuscripts to be published.